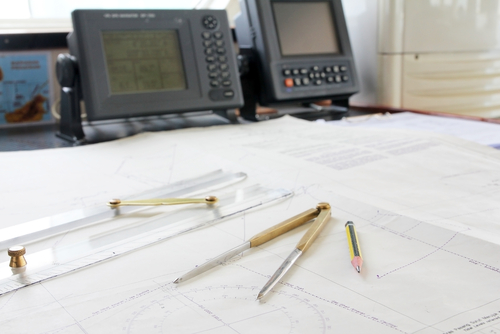
How to work out your waiting time trajectories accurately
06/10/2016by Rob Findlay
All over the NHS in both England and Scotland, managers are being asked to produce trajectories for reducing the number (or proportion) of long-waiters on their waiting lists. The people who request this information probably have no idea how difficult it is to do these calculations properly, and it is common for analysts and managers to submit trajectories that are little more than educated guesses (or straight lines that only just achieve the target).
Nevertheless trajectories are a sensible thing to ask for, especially when targets are not currently being met and a recovery plan is needed. Good planning is a powerful aid to good management. So here I will outline the steps in the calculations if you are attempting this with a reasonable level of accuracy in a spreadsheet model.
As you can already see from the length of this post, there is lot involved. So why not use Gooroo Planner instead? It is an advanced and mature model that is tried and trusted across the NHS, and capable of great accuracy. It can also be automated so that you don’t even have to run it (let alone build it). If you’d like to learn more, then please get in touch.
Producing a trajectory is rarely the end of the story, of course. You may then need to run alternative scenarios, or phase different specialties to achieve different targets by different deadlines, or to future-proof those targets by planning for them to be sustained continuously thereafter. But for now let’s stick to the more straightforward scenario.
But first: weeks or months? In a financially-led process you will normally be asked for monthly trajectories, but you will also want to make comparisons against the capacity you have available, and capacity works in weeks. So it is better to plan in weeks, and then convert to months at the last minute to feed the financially-led process.
The task breaks down into several steps:
Demand
The first and most important step is to work out the amount of demand that needs to be met every week. To do this, you need a way of estimating demand that is net of those patients who are removed without treatment, so that you can make direct comparisons between demand and activity.
Unfortunately the most direct method of calculating net demand is unreliable, because it uses data that is commonly inaccurate (additions to the waiting list, and removals for reasons other than treatment).
Fortunately there is a better method which is approximately activity plus the change in waiting list size. For a more exact result you also need to make a small adjustment to account for patients who are cancelled and removed at short notice. The formula is derived in the attached document.
This method is better because it mainly relies on activity, which is almost always the most accurate data in the system. Although it also relies on waiting list sizes which are often unreliable, any errors are usually systematic, which means that changes in the waiting list (which are what the calculations actually use) are usually pretty accurate.
The main exception to this rule is when there has been a major one-off validation exercise, but even when that happens it is usually better to adjust the initial waiting list snapshot as if it had also been validated, than to switch to the alternative and less-reliable method of additions minus removals.
Having established the formula for calculating demand, you also need to know how demand varies through the year so that you can work out the week by week demand. You may also wish to inflate demand over time in line with any trend demand growth that you are expecting.
Activity
Work out the amount of activity that is likely to be done every week. If you have time, you could ask operational managers for their plans, or ask them to agree to deliver plans that you have calculated to achieve the waiting time targets.
But it might be faster and more accurate simply to use the activity that has historically been done each week. Averaging over a three year period is usually a pragmatic way of obtaining this.
Waiting list
Take the number of patients that are currently on the waiting list, add the weekly demand as calculated above and subtract the weekly activity, and that gives you approximately the number of patients you are expecting to find on the waiting list at the end of every week. Again, the exact calculation would also take into account patients who are cancelled and removed at short notice, as detailed by the formulae in the attached document.
Any errors in the previous steps, particularly around patients removed for reasons other than activity, will be magnified as soon as you focus on the waiting list, which is why it is so important to use a method for calculating demand that is resilient to common data errors.
Long waiters
The final step is to extract the number of long-waiting patients from the total number of patients on the waiting list. This is very challenging, because some patients are clinically urgent and therefore treated out of turn, as well as some other patients; some patients are removed or cancelled which further disrupts patient scheduling and raises waiting times; and the patient scheduling tactics in force will also affect the number of long waiters.
In practice most people fudge these calculations by using a rule of thumb, which unfortunately magnifies any existing data errors and introduces significant further inaccuracy. Before discussing an accurate approach I will outline some of these cruder methods.
One common approach is to calculate the simple clearance time, or mean average waiting time according to Little’s Law. This is the size of the waiting list divided by the rate of activity; for instance if there are 100 patients on the waiting list, and patients are treated (and removed for other reasons) at the rate of 10 per week, then the mean average waiting time is 10 weeks. The biggest problem with this approach is that real waiting lists are a mixture of patients with different clinical urgencies. If half the patients are clinically urgent and need to jump the queue, then the other half will wait longer. The whole point of the long-wait trajectory we are trying to calculate is to reveal the number who will wait longer, but the average waiting time calculation does not help with this. A common workaround is to try to allow for this by multiplying the mean average by some factor to estimate how long routine patients will wait, but this is a very rough method and the factor required will differ greatly from service to service.
An alternative approach is to separate the casemix into urgent and routine, and perform waiting time calculations on the routine element only. This gives a closer result, but is still inaccurate because it neither reflects how patients are scheduled in real life, nor takes account of other forms of disruption or the effect of patient scheduling tactics. It also has the practical drawback that clinical urgency is not always consistent in the raw data as patients pass through the waiting list.
Drawing a simple geometrical waiting list shape is an approach that I worked up in the mid 1990s, and it proved useful in its day, mainly because it took direct account of the effect of clinical priorities on the waiting list shape. However it still involved large and arbitrary assumptions about the management of non-urgent patients, and did not directly take account of the disruption caused by things like removals, random variations, or different scheduling tactics. So it helpfully pointed to the theoretical potential to reduce waiting times by improving patient scheduling, but it did not show to what extent such changes would be realistic, or what actions were needed to make it happen.
So how can we do the job more accurately? We need to take proper account of the key influencers on longer waiters, including: the addition rate, list size, clinical priorities, removal rate, and patient scheduling methods being used. In the absence of a full theoretical basis for calculating this, a suitable approach is to simulate the patient scheduling process using a discrete event simulation model, and measure the waiting times and long waiters that arise.
Unfortunately this is time consuming, and because the simulation is driven by random events it needs to be repeated many times (often over centuries of simulated clinical practice) to produce a reliable indication of the typical behaviour of just one scenario. It would not be practical to do this every time we wanted to calculate a waiting time or the expected number of long waiters.
In practice we need to study the behaviour of the simulator under a wide range of scenarios, to determine an empirical formula which describes its behaviour more generally. That means more work up-front (in my experience it is a multi-year research project in its own right) but at the end you will have a formula for long waiting times that can produce reliable results very quickly. Where necessary, those results can be substantiated using simulation if there are questions about the accuracy of the calculation.
But even this approach does not necessarily reflect real life long waits accurately. To mangle Tolstoy: every well managed waiting list is alike, but every badly managed waiting list is badly managed in its own way. A discrete event simulation model will reveal the behaviour of a waiting list that is managed (hopefully well-managed) in a particular way, but no such model can take account of all the human behaviours and feedbacks that exist in the unfortunately common situation when real-life waiting lists are managed to a target instead of the needs of patients. Some adjustment may still be necessary to allow for real waiting list management being suboptimal, which can be done by looking at the data and making suitable adjustments to the urgency rates and expected waiting times in the formula.
Designing the model
Once you have all the necessary formulae in place, you will need to repeat the calculations separately for every service in the hospital because each will have different waiting time pressures. Scaling the model up presents its own challenges, and now it is time to consider the layout of the spreadsheet model.
The trickiest bit is modelling along clinical pathways. The principles are straightforward enough: if you want to calculate the number of long-waiting surgical inpatients and daycases, then your plans need to take account of how patients move along the clinical pathways, and the knock-on effects that changing outpatient activity (for instance) will have on the demand for surgery over time. A typical cancer pathway is even more complicated and typically contains multiple diagnostic stages and treatment options.
A common way to calculate along clinical pathways is to measure the conversion rate from one stage of the pathway to the next, and multiply any non-recurring activity upstream by the same conversion rate to work out the extra demand downstream. A simpler and equivalent method is to assume that if upstream activity at one stage of the pathway increases by (say) 5 per cent, then downstream demand at the next stage of the pathway also increases by 5 per cent.
However it is challenging in practice to structure a spreadsheet model around clinical pathways, because spreadsheets are two dimensional. Usually one dimension is used to lay out the steps in the calculations, and the other to lay out the different clinical services in the model. Clinical pathways are interrelationships between services that step outside this structure, so they typically need to be created manually between different parts of the spreadsheet model. Those interrelationships are sometimes bespoke to a particular clinical pathway, which means that care is needed when adapting the model manually for a different clinical pathway. It also means that the spreadsheet model cannot always be extended simply by copying blocks of cells.
A further consideration when modelling in a spreadsheet is size. Even relatively naïve spreadsheet models have a tendency to grow enormously, and this is especially true of spreadsheets that produce trajectories over time across all the clinical services in a hospital. One such model which was doing the rounds in the NHS a few years ago had over 100,000 active cells in it, and after some weeks of effort the information department we were talking to had given up trying to populate it.
Research into spreadsheet modelling further shows that even carefully-constructed spreadsheets typically have errors in at least one per cent of formula cells, so a large model can be expected to be riddled with them. For instance, cutting instead of copying cells before pasting is a common cause of error in established spreadsheets.
The result
Once the model has been constructed, the clinical pathways set up, and after demand and activity have cascaded down the clinical pathways over the time period being modelled, you can add up the number of long waiters to get your projected total.
How close will your projections turn out?
Depending on the skill of your model, and with a bit of luck, you could get pretty close – and precision trajectory planning has been achieved using these methods (though using Gooroo Planner rather than a spreadsheet).
It isn’t all about the accuracy of the result – a good model should help you understand as well as predict – but accuracy certainly gives assurance that your methods are correct as well useful.
“I need our long-wait trajectories for a meeting tomorrow!”
It’s hard enough coming up with monthly planning trajectories when you have several weeks to do it in. Now imagine having to do it for tomorrow.
Not only did one Scottish Health Board manage it, but their plans turned out to have pinpoint accuracy as the months unfolded.
Return to Post Index
Leave a Reply
You must be logged in to post a comment.