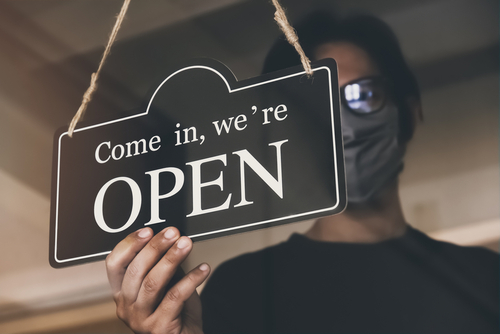
Covid-19 planning part 2 – the recovery
15/07/2020by Rob Findlay
This is a live technical document about planning the post-covid recovery in Gooroo Planner, and was last updated on 15th July 2020. (Modelling the elective shutdown was covered in a previous post.)
If you have any comments or questions please email me directly (rob.findlay@gooroo.co.uk) so that I can update this document.
When planning the post-covid recovery there are a number of things to consider, under the broad headings of demand, activity, capacity and waiting list:
- What will the level and pattern of demand look like (allowing for delayed or lost referrals, complex late presentations, non-electives)?
- How fast will the recovery be (how much activity will be done)?
- How will capacity be organised (virtual clinics, covid/non-covid split, site reorganisations, system-wide working)?
- What is the starting point (how much of the current waiting list will actually need treatment, what about delayed follow-ups)?
Demand
The simplest modelling scenario is that post-covid demand will be the same as pre-covid demand, because then you can simply upload data from the pre-covid period, and Gooroo Planner will measure that rate of demand and project it forward into the future. My understanding is that this is the default assumption in some areas.
If you want to assume something different, then there are three main ways of doing this.
The first and simplest way is to use the Growth field, which applies a trend to the demand for each service.
For instance Growth of 0.05 means that demand is expected to grow by 5 per cent annually (and -0.1 means demand is expected to shrink by 10 per cent annually). Growth is applied compound, so after one year demand would be 105% (or 90%) of its inital level, and after two years it would be 110% (or 81%).
Growth is ideal for genuine trends. It can also be a useful way of making ballpark estimates about other effects over the coming year, but it is probably a bit crude for covid-induced baseline changes.
The second, more granular, method changes the demand for each service by an absolute amount, by using PastExtraDem to adjust the measured demand for each service during the past period, or FutExtraDem to adjust for expected changes in future.
This could be used to account for referrals that are expected to be lost permanently, or for surges as delayed referrals come back in, or for new kinds of demand such as remote support for primary care.
For instance PastExtraDem of -200 means that about 200 of the past period demand is not expected to recur in future, and FutExtraDem of 50 could be used to mean that 50 patients whose referral was held back during shutdown will be referred soon. This is useful for estimating the overall impact if you have an idea of the absolute changes you are expecting.
The third, and most detailed, approach is to rewrite the past, so that it matches the expected future. This would be done at patient level in your data queries, and should if possible be consistent across all the files (activity, additions, waiting list snapshots and statistics).
For instance, if you expect certain clinic codes to be handled in primary care in future, and not be part of your demand, then you could exclude from all your data any patients who were listed under those clinic codes.
All these scenarios use pre-covid data as the baseline, and for post-covid modelling purposes this will probably be reasonable for several months to come.
Activity
There are two possible approaches when it comes to activity. You can either start by deciding how much activity you think you can deliver, and then work out the consequences. Or you can start by deciding the outcome you want to achieve, and then work out what activity is needed to deliver it.
In practice, you will probably need to do both, and in that order.
For the next several months (perhaps until winter is over) activity will be constrained by covid. You will want to estimate the activity you can deliver, and set your activity plan accordingly using the FutActiv field and selecting the “Use data provided (FutActiv)” activity scenario. The software will then work out the consequences for waiting lists and waiting times.
After that, you may be on a recovery trajectory towards shorter waiting times. You can roll your first model forwards within Gooroo Planner (using the ‘Convert to dataset’ icon in the Report manager), update it with the waiting times you intend to achieve at the end of your chosen multi-year timescale (using a high level assumptions table), check that the “Match waiting list targets” activity scenario is selected, and then Gooroo Planner will work out the activity and capacity you need to deliver that objective.
You can even go further, and ask Gooroo Planner to clear back the waiting list to the point where your targets can then be met every week of the year, with waiting times rising and falling naturally through the seasons but never breaching. Again this is straightforward to model and this short video shows how.
Capacity
Capacity is usually the most complicated part of demand and capacity planning, not because beds, theatres and clinics are hard to calculate, but because actual physical capacity tends to be surprisingly difficult to measure on a basis that is directly comparable with your activity data.
One way to sidestep this problem is not to calculate capacity at all, and simply calculate activity instead. This is a popular approach when it comes to clinics, for which a slot count is often as useful as hours per week. But this simple approach may not be necessary for beds or theatres where the available capacity tends to be easier to measure.
The next issue is: what level of detail should we be modelling at? In normal times the answer is the level of inflexibility between services, which is usually specialty level, except in highly-subspecialised services such as Orthopaedics where sub-specialty is preferred.
However in the post-covid recovery we also need to consider new ways of working such as site reconfigurations, virtual clinics, and covid/non-covid splits.
Bed occupancy
It is widely recognised that bed occupancy was too high in the pre-covid NHS, so that hospitals ran out of non-elective beds too often, with patients suffering the clinical risks associated with being on the wrong ward and of having their surgery cancelled.
The traditional method for calculating beds relies on a blanket assumption about bed occupancy (the proportion of beds that are occupied on average). The most popular numbers are 85 per cent, or 92 per cent in England. However a moment’s reflection is enough to realise that no number can possibly be right everywhere – smaller bed pools are more vulnerable to variation and will require lower bed occupancy, and vice versa for larger bed pools.
It is now possible to calculate the right number of beds for each specific bed pool, based on the clinically acceptable risks of non-elective patients overspilling into different parts of the hospital. The method is explained fully in NHS England and NHS Improvement ECIST guidance here, in a speech to the Royal College of Emergency Medicine here, and you can find more details of running the calculations for your hospital using Gooroo Beds here.
Site reconfigurations
For site reconfigurations, the easiest approach is usually to lump all the sites together, work out what is needed overall, and then split the activity and capacity into more detail afterwards. For instance, you may want to work out what is needed (by specialty, by stage of treatment) across an entire health system, and then divide the work between the hospital sites afterwards.
(If you are planning across an entire health system then you will face the additional task of getting all the necessary data system-wide. A similar task faces you if the system is moving to a system-wide waiting list. You could extract data from each Trust separately and then merge it into Gooroo Planner. A more stable long-term solution would be to merge all the source data tidily into a single unified data layer, using Insource’s Health Data Enterprise which Gooroo Planner already connects to. Please contact Insource directly if you would like to explore that option. )
New points of delivery
For virtual clinics and other novel points of delivery, you could follow the approach above, but it may be better to split the data up according to the expected future operating model. This is the same as the “rewrite the past, so that it matches the expected future” approach that we used for demand above.
For instance, if certain clinic codes will be delivered virtually in future, then all patients in your past data who were assigned to those clinic codes can be streamed to a new virtual ‘activity type’ under the HeadType header.
Time taken for infection control
You may want to reflect the extra time taken to don and doff PPE and enact other infection control measures. Gooroo Planner enables this in both the theatre and clinic calculations. I’ll explain it here with reference to theatres, and you can find more details and the clinic equivalents in the Statistical Dataset section and the Results section of the Gooroo Planner documentation.
There are two ways of reflecting lost time when calculating theatre capacity. One is the changeover interval (FutThtChg) to reflect per-case delays, and this will have greater impact on services with many short cases. The other is the familiar utilisation rate (FutThtUtil) to reflect the fact that sessions cannot be packed and utilised 100%.
These two numbers are combined when calculating theatre times, as you can see in the documentation where the theatre time calculations are explained. The formulae simplify to:
theatre time per week =
theatre activity per week * (time per patient + changeover interval) / utilisation
So you could overwrite the utilisation figure which Gooroo Planner has measured automatically from your theatre data, with a lower figure that reflects the post-covid situation better. Or you could leave utilisation alone, and use the changeover interval to reflect the situation and the greater effect it will have on lists with many short cases. Or you could combine the two in some way, although that might be more difficult to explain to others.
If you want to see how your chosen changeover intervals and utilisation rates would look, if you converted them into pure changeover or pure utilisation, we provide two results fields which do that:
ResThtEquivChg (Equivalent theatre changeover time at full utilisation)
ResThtEquivUtil (Equivalent theatre utilisation at zero changeover time)
Waiting list
The good news is that you probably don’t need to take the trouble to calculate the post-shutdown waiting list using Gooroo Planner, because the shutdown has (largely) already happened, and you can simply upload the latest waiting list (with a census date to match the start of your future period).
The bad news is that the waiting list itself is open to question. Some patients may be returned to primary care after review. Some patients, who would already have been referred in normal times but were not because of the shutdown, may be referred soon in a post-covid surge. And patients with delayed follow-ups should, according to section 5.1.2 of the English RTT guidance, now be on the RTT waiting list; although the move to Patient Initiated Follow Up suggests that many will no longer have pending appointments.
When you have quantified the above assumptions, you then have a choice about how to reflect them in your Gooroo Planner modelling.
You can either increase or decrease the waiting list at the start of the future period (by changing FutWLStart to a new value), or you can increase or decrease future demand (using FutExtraDem). The former is probably easier if you have an absolute waiting list size in mind, the latter if you want to apply a particular change to the list size.
Support
If you have a Gooroo Planner licence, please get in touch if you need support.
If you are interested in using Gooroo Planner or Gooroo Beds, please get in touch for a free (virtual) demo.
Return to Post Index
Leave a Reply
You must be logged in to post a comment.